
Ultimate Guide to AI Technologies in Fall Prevention
Importance of Fall Prevention
Falls among seniors have become a healthcare emergency hiding in plain sight. The numbers tell a stark story: 36 million falls annually in the US lead to nearly 39,0001 deaths and send 3 million older Americans to emergency departments. When one in five2 of these falls results in serious trauma like broken bones or head injuries, the human cost is devastating—and the financial impact reaches $50 billion yearly, with Medicare and Medicaid bearing 75% of the burden3.
This crisis is set to deepen. By 2040, global costs from fall injuries are projected to surge to $240 billion4. Meanwhile, US healthcare facilities face mounting pressure—failing to meet fall prevention requirements in the Inpatient Quality Reporting Program can trigger significant Medicare reimbursement penalties5.But there's hope. AI-powered fall prevention technology offers a transformative solution, helping healthcare providers protect patients, control costs, and maintain regulatory compliance. Let's explore how these innovative technologies are reshaping fall prevention across the healthcare spectrum.
But there's hope. AI-powered fall prevention technology offers a transformative solution, helping healthcare providers protect patients, control costs, and maintain regulatory compliance. Let's explore how these innovative technologies are reshaping fall prevention across the healthcare spectrum.
Overview of AI Technologies in Fall Prevention
The healthcare industry faces a critical challenge in fall prevention. While traditional bed alarms are ineffective and patient sitters are costly, a new wave of AI-powered solutions promises better outcomes. Today, over 50 different systems compete for attention, each claiming to help caregivers deliver more timely and proactive assistance.
Look beneath the surface, though, and you'll find these solutions rely on a surprisingly small set of core technologies6. From computer vision and audio analysis to radio frequency detection and thermal sensing, each approach has its own strengths and limitations.
This guide cuts through the complexity. We'll start by examining the science behind these fundamental sensing technologies, then evaluate how leading solutions implement them in real-world healthcare settings. Whether you're considering your first AI fall prevention system or looking to upgrade existing technology, understanding these basics is crucial for making informed decisions. Let’s begin with video which we are all very familiar with.

Video Technology

AI-powered cameras watch people's movements in real-time, like a smart security system. The AI analyzes how someone walks and moves, checking for signs they might fall – like wobbling or unsteady steps. If it spots risky movement, it instantly alerts caregivers who can help prevent a fall.
Using videos, key body points (like joints and limbs) can be identified and tracked how they move. Algorithms can compare these movements to thousands of examples it learned from, identifying patterns that signal fall risks – like unsteady walking or sudden balance shifts.
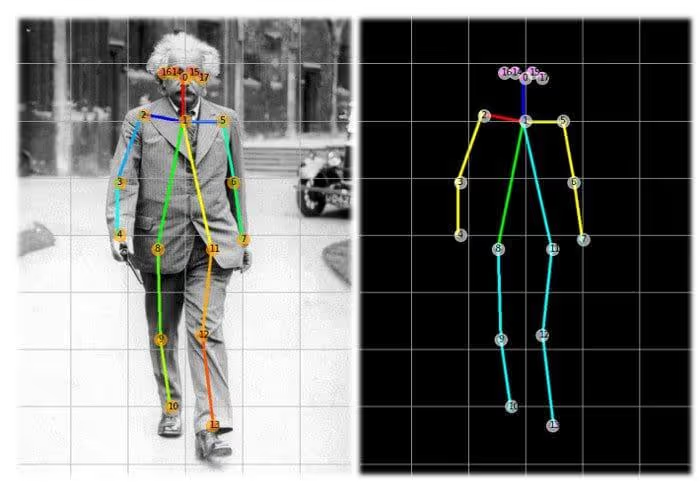

Ultrasound Sensor

Ultrasonic sensors work like nature's own sonar system. Think of a bat navigating through dark caves – it emits high-frequency sound waves that bounce off cave walls and return, helping it build a "sound map" of its surroundings. Ultrasonic fall prevention systems use the same principle, but with much more precision.
These sensors emit high-frequency sound waves (beyond human hearing range) that bounce off a person's body and return to the sensor. Just as your echo returns faster from a nearby canyon wall than a distant one, these sound waves return at different speeds depending on their distance from various body parts. The system measures these timing differences to create a detailed "sound image" of a person's position and movement in the room.
Radio Frequency Sensor
Radio Frequency (RF) sensors work like a miniature radar system. Just as radar tracks airplanes by bouncing radio waves off them, RF sensors emit low-power radio waves that reflect off the human body. These reflections create a unique pattern based on a person's position, and movement as shown below7.

Think of throwing a pebble into a still pond – the ripples change when they hit objects in the water. Similarly, RF waves change when they interact with a person, and these changes tell us about their location and motion. The system can detect subtle movements like chest rise from breathing when someone is still, or larger motions like sitting up or attempting to stand.
Passive Infrared (PIR) Thermal Sensor

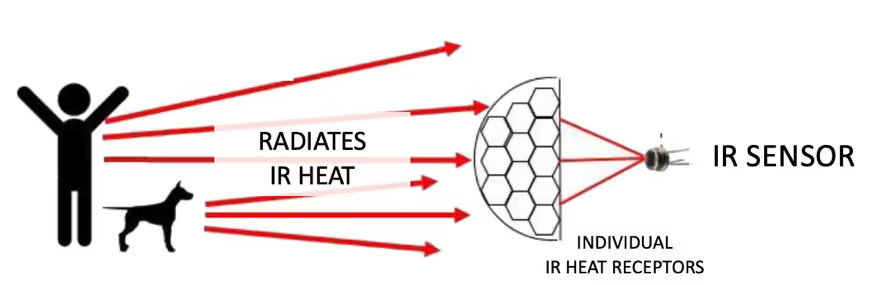

Infrared (IR) sensors work like thermal cameras. The sensor is able to distinguish hundreds to several thousand different temperatures of the objects that naturally give off IR heat. It uses this to creates heat map of the objects. Just as nighttime wildlife cameras can spot warm-blooded animals in complete darkness, IR sensors create a heat map of people in a room by detecting their body temperature against the cooler background. The sensor can distinguish between a person sitting in bed, standing up, or leaving the bed entirely based on how the heat pattern changes.
LiDAR Sensor
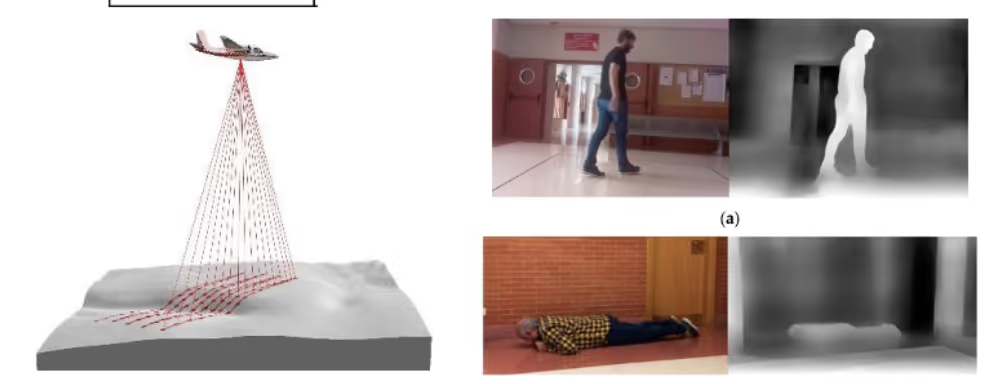
LiDAR (Light Detection and Ranging) creates highly detailed 3D maps by emitting millions of laser pulses per second. The system measures the precise time each light pulse takes to bounce back after hitting objects, calculating distances with millimeter accuracy. LiDAR can build richly detailed point clouds that capture exact shapes and positions of people and objects in a room.
Accelerometer Motion Sensor


An accelerometer tracks motion by measuring changes in velocity and orientation across three axes (X, Y, Z). When attached to a person or device worn by them, it can send out signals in real-time and software can be used to understand:
- Position changes (lying down, sitting, standing)
- Movement speed and direction
- Sudden motions
- Activity patterns and intensity of movement
Think of it like a bubble level used in construction - when you tilt the level, the bubble moves to show orientation. Similarly, an accelerometer uses tiny internal sensors (invisible to the naked-eye) to detect how it's being moved or tilted, allowing it to track a person's movements in real-time.
Definition of Fall Prevention
According to the Agency for Healthcare Research and Quality (AHRQ), fall prevention requires a multi-faceted approach, including:
- Identifying individual fall risks through assessments
- Implementing environmental modifications (e.g., non-slip flooring)
- Utilizing clinical interventions such as medication management
- Providing assistive devices as needed
- Educating patients on fall prevention strategies
This approach focuses on both proactive measures to reduce fall risk and responsive interventions when necessary.
For decades, acute and post-acute healthcare organizations have worked to comply with AHRQ recommendations. Many commercially available systems provide tools and insights to help clinicians make informed decisions and proactively mitigate fall risk. However, a critical gap remains in real-time, responsive intervention to actively prevent falls.
Commercial fall prevention systems vary significantly in technology, response time, and overall effectiveness in preventing falls. The table below highlights noteworthy AI-driven fall prevention and detection technologies, evaluating their capabilities, costs, and ability to prevent falls.
Among these solutions, OK2StandUP stands out as it directly addresses the unmet need for real-time responsive intervention, filling a crucial gap in fall prevention.
Solution
SENSOR TECHNOLOGY
Video
X
X
X
X
X
X
X
X
X
X
INFERARED
X
X
X
X
X
X
X
X
X
X
RDAR
x
x
x
x
x
Table
Table
Table
X
Table
LiDER
x
x
x
x
x
Table
X
Table
Table
Table
ACCELEROMETER
x
x
x
x
x
Table
Table
X
Table
Table
Solution
Solution
VIRTUAL OBSERVER
REQUIRED
No
No
NO;
IMAGES RECORDED
YES;
IMAGES RECORDED
YES;
IMAGES RECORDED
YES;
IMAGES RECORDED
YES; I
MAGES RECORDED
No
No
YES;
IMAGES RECORDED
AI TIME TO REQ’D TO ALERT OFINTENTION
< 6 sec
30-65 sec
30-90 sec
OBSERVER
DISPATCH
VIRTUAL OBSERVER
AFTER
FALL
AFTER
FALL
AFTER
FALL
AFTER
FALL
AFTER
FALL
LOCATION
ANY
WHERE
ROOM
ROOM
ROOM
ROOM
ROOM
ANY
WHERE
ANY
WHERE
ROOM
ROOM
Solution
Solution
VIDEO LINKS
VIDEO1
VIDEO2
VIDEO 1
VIDEO1
VIDEO2
VIDEO1
VIDEO2
VIDEO1
VIDEO1
Table
VIDEO1
VIDEO2
VIDEO1
VIDEO2
VIDEO1
FDA REGISTERED
Yes
No
No
No
Yes
Table
Yes
No
No
Yes
COST RANGE
LOW TO MODERATE
MODERATE TO HIGH
MODERATE TO HIGH
HIGH
HIGH
HIGH
HIGH
MODERATE
MODERATE
HIGH
Solution
SENSOR TECHNOLOGY
Solution
Solution
Video
X
X
X
X
X
X
X
X
X
X
INFERARED
X
X
X
X
X
X
X
X
X
X
RDAR
x
x
x
x
x
Table
Table
Table
X
Table
LiDER
x
x
x
x
x
Table
X
Table
Table
Table
ACCELEROMETER
x
x
x
x
x
Table
Table
X
Table
Table
VIRTUAL OBSERVER
REQUIRED
No
No
NO;
IMAGES RECORDED
YES;
IMAGES RECORDED
YES;
IMAGES RECORDED
YES;
IMAGES RECORDED
YES; I
MAGES RECORDED
No
No
YES;
IMAGES RECORDED
AI TIME TO REQ’D TO ALERT OFINTENTION
< 6 sec
30-65 sec
30-90 sec
OBSERVER
DISPATCH
VIRTUAL OBSERVER
AFTER
FALL
AFTER
FALL
AFTER
FALL
AFTER
FALL
AFTER
FALL
LOCATION
ANY
WHERE
ROOM
ROOM
ROOM
ROOM
ROOM
ANY
WHERE
ANY
WHERE
ROOM
ROOM
VIDEO LINKS
VIDEO1
VIDEO2
VIDEO 1
VIDEO1
VIDEO2
VIDEO1
VIDEO2
VIDEO1
VIDEO1
Table
VIDEO1
VIDEO2
VIDEO1
VIDEO2
VIDEO1
FDA REGISTERED
Yes
No
No
No
Yes
Table
Yes
No
No
Yes
COST RANGE
LOW TO MODERATE
MODERATE TO HIGH
MODERATE TO HIGH
HIGH
HIGH
HIGH
HIGH
MODERATE
MODERATE
HIGH
Ability to Prevent Falls
Fall prevention technology solutions can be categorized into four groups with the legacy solutions on the bottom left and working towards real-time fall prevention on the top right. Ultimately, for effective fall prevention, technology providers must empower frontline caregivers to intervene in a timely manner without adding to their workload.
The graph below illustrates these four categories for better visualization.
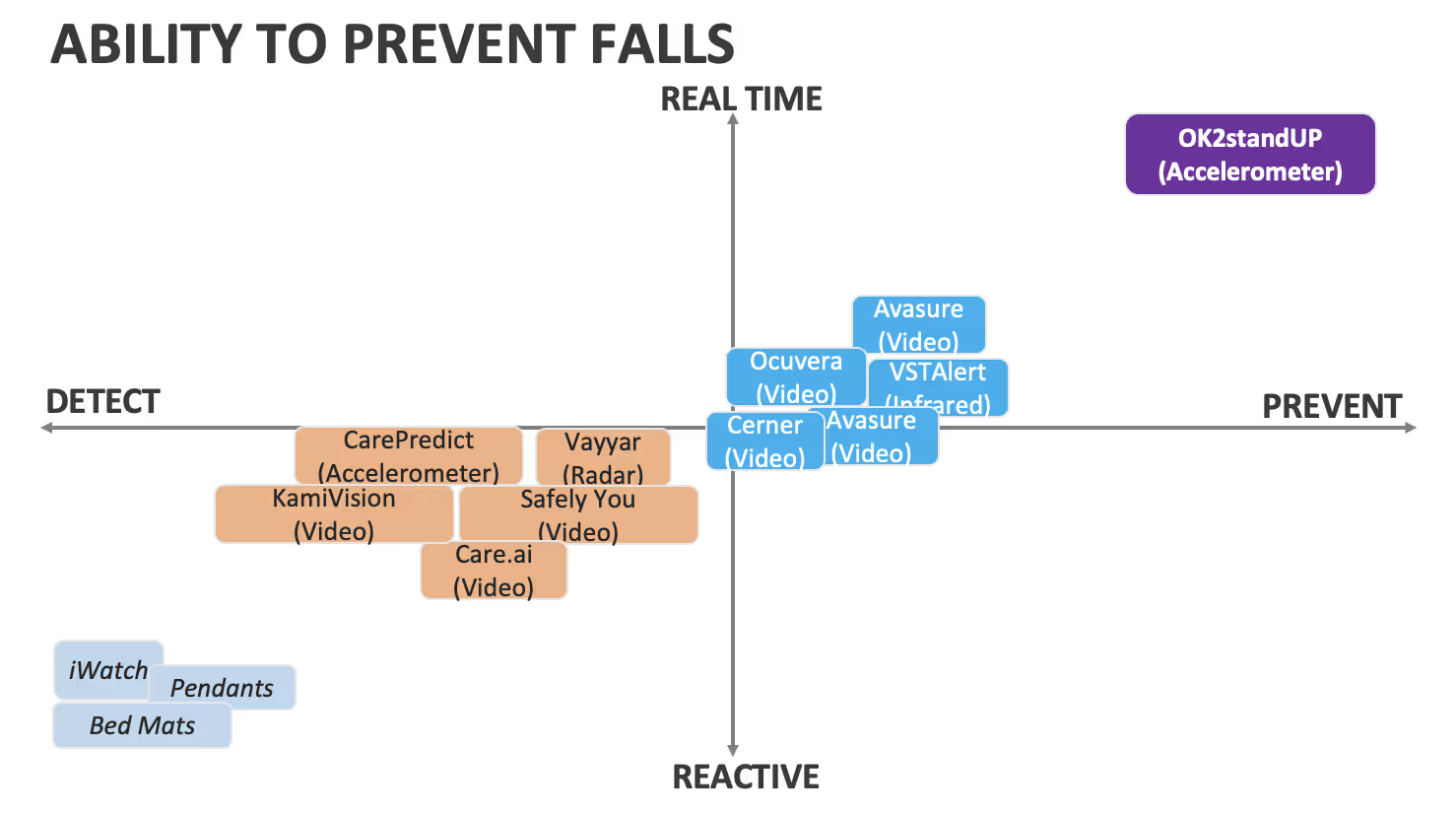
Responsibilities and Legal Risks of Video-Based Fall Prevention Systems
Video monitoring systems offer tremendous value by providing high-fidelity documentation of falls, which can then be reviewed to enhance care delivery and improve health outcomes. However, the use of video in fall prevention raises important questions regarding an organization's responsibilities and legal risks, particularly concerning privacy laws, regulatory compliance, and institutional policies. Below is a detailed breakdown of these considerations.
Responsibility for Using Video to Prevent Falls
Video monitoring in fall prevention can take different forms. Traditional video systems capture identifiable images of individuals, while technologies such as infrared (IR), radio frequency (RF), or LiDAR generate silhouette-based recordings. The level of responsibility an organization assumes when using video for fall prevention depends on several factors, including:
- How the video is utilized in clinical decision-making (e.g., real-time monitoring, stored for later analysis, or used for staff training).
- Whether staff members are expected to actively monitor the video feed and intervene when a fall risk is detected.
- Hospital policies and industry best practices regarding patient monitoring.
To ensure clarity and compliance, organizations should establish:
- Whether video monitoring is passive (recorded for later review) or active (requiring real-time staff intervention).
- Expectations for nursing staff when fall risk behaviors are observed.
- Defined protocols for responding to incidents captured on the video feed.
By addressing these considerations, healthcare organizations can maximize the benefits of video-based fall prevention while mitigating potential legal actions which is a significant source of lawsuits against healthcare facilities9 and ethical risks.
A Personal Note from Dr. Yang

Fall prevention technology isn’t just about detecting risks—it’s about empowering caregivers to act before a fall occurs. At OK2StandUP, we remain steadfast in our mission to close the gap in responsive interventions and create safer environments for everyone. Thank you for taking the time to read about this important work. Together, we can make a meaningful difference in patient safety.
Warm regards,
Dr. Yang
CEO
OK2StandUP
References
1. https://www.cdc.gov/mmwr/volumes/72/wr/mm7235a1.htm
2. https://pubmed.ncbi.nlm.nih.gov/36210739/
3. https://pmc.ncbi.nlm.nih.gov/articles/PMC6089380/
7. https://ieeexplore.ieee.org/abstract/document/10132105
8. https://www.ahrq.gov/patient-safety/settings/hospital/fall-prevention/toolkit/index.html
9 Healthcare Journal, 2023 Apr 30;11(9):1290. doi: 10.3390/healthcare11091290
For more information about any of these technologies, feel free to reach out to me at eunice@ok2standup.com.
Subscribe to our newsletter
We're committed to your privacy. OK2StandUP uses the information you provide to us to contact you about our relevant content, products, and services. You may unsubscribe from these communications at any time. For more information, check out our privacy policy.
